Ok I have figured this out. It was to do with the order of prediction classes provided to roc_curve()
. Here is a reproducible example below.
library(tidymodels)
library(dplyr)
dfr <- iris %>% mutate(Species=factor(Species))
data_split <- initial_split(dfr,prob=0.70,strata=Species)
data_train <- training(data_split)
data_test <- testing(data_split)
data_tune_cv <- vfold_cv(data_train,v=10,repeats=1,strata=Species)
recipe <- data_train %>% recipe(Species ~ .)
rf_spec <- rand_forest(mtry = tune(), min_n = tune(), trees = tune()) %>%
set_mode("classification") %>%
set_engine("ranger", num.threads = (parallel::detectCores()-1), importance = "impurity")
rf_wf <- workflow() %>%
add_recipe(recipe) %>%
add_model(rf_spec)
rf_metrics <- metric_set(roc_auc,accuracy,bal_accuracy,f_meas,kap,mcc,precision,recall)
rf_grid <- grid_latin_hypercube(mtry=finalize(mtry(),data_train), min_n(), trees(), size=20)
rf_tune <- tune_grid(rf_wf, resamples=data_tune_cv, grid=rf_grid)
#> ! Fold01: preprocessor 1/1, model 1/20: 5 columns were requested but there were 4 predictors in the data. 4 will...
#> ! Fold01: preprocessor 1/1, model 5/20: 5 columns were requested but there were 4 predictors in the data. 4 will...
#> ! Fold01: preprocessor 1/1, model 17/20: 5 columns were requested but there were 4 predictors in the data. 4 will...
#> ! Fold02: preprocessor 1/1, model 1/20: 5 columns were requested but there were 4 predictors in the data. 4 will...
#> ! Fold02: preprocessor 1/1, model 5/20: 5 columns were requested but there were 4 predictors in the data. 4 will...
#> ! Fold02: preprocessor 1/1, model 17/20: 5 columns were requested but there were 4 predictors in the data. 4 will...
#> ! Fold03: preprocessor 1/1, model 1/20: 5 columns were requested but there were 4 predictors in the data. 4 will...
#> ! Fold03: preprocessor 1/1, model 5/20: 5 columns were requested but there were 4 predictors in the data. 4 will...
#> ! Fold03: preprocessor 1/1, model 17/20: 5 columns were requested but there were 4 predictors in the data. 4 will...
#> ! Fold04: preprocessor 1/1, model 1/20: 5 columns were requested but there were 4 predictors in the data. 4 will...
#> ! Fold04: preprocessor 1/1, model 5/20: 5 columns were requested but there were 4 predictors in the data. 4 will...
#> ! Fold04: preprocessor 1/1, model 17/20: 5 columns were requested but there were 4 predictors in the data. 4 will...
#> ! Fold05: preprocessor 1/1, model 1/20: 5 columns were requested but there were 4 predictors in the data. 4 will...
#> ! Fold05: preprocessor 1/1, model 5/20: 5 columns were requested but there were 4 predictors in the data. 4 will...
#> ! Fold05: preprocessor 1/1, model 17/20: 5 columns were requested but there were 4 predictors in the data. 4 will...
#> ! Fold06: preprocessor 1/1, model 1/20: 5 columns were requested but there were 4 predictors in the data. 4 will...
#> ! Fold06: preprocessor 1/1, model 5/20: 5 columns were requested but there were 4 predictors in the data. 4 will...
#> ! Fold06: preprocessor 1/1, model 17/20: 5 columns were requested but there were 4 predictors in the data. 4 will...
#> ! Fold07: preprocessor 1/1, model 1/20: 5 columns were requested but there were 4 predictors in the data. 4 will...
#> ! Fold07: preprocessor 1/1, model 5/20: 5 columns were requested but there were 4 predictors in the data. 4 will...
#> ! Fold07: preprocessor 1/1, model 17/20: 5 columns were requested but there were 4 predictors in the data. 4 will...
#> ! Fold08: preprocessor 1/1, model 1/20: 5 columns were requested but there were 4 predictors in the data. 4 will...
#> ! Fold08: preprocessor 1/1, model 5/20: 5 columns were requested but there were 4 predictors in the data. 4 will...
#> ! Fold08: preprocessor 1/1, model 17/20: 5 columns were requested but there were 4 predictors in the data. 4 will...
#> ! Fold09: preprocessor 1/1, model 1/20: 5 columns were requested but there were 4 predictors in the data. 4 will...
#> ! Fold09: preprocessor 1/1, model 5/20: 5 columns were requested but there were 4 predictors in the data. 4 will...
#> ! Fold09: preprocessor 1/1, model 17/20: 5 columns were requested but there were 4 predictors in the data. 4 will...
#> ! Fold10: preprocessor 1/1, model 1/20: 5 columns were requested but there were 4 predictors in the data. 4 will...
#> ! Fold10: preprocessor 1/1, model 5/20: 5 columns were requested but there were 4 predictors in the data. 4 will...
#> ! Fold10: preprocessor 1/1, model 17/20: 5 columns were requested but there were 4 predictors in the data. 4 will...
rf_best <- select_best(rf_tune,metric="roc_auc")
rf_training_pred <- rf_wf %>%
finalize_workflow(rf_best) %>%
fit_resamples(data_tune_cv,control = control_grid(save_pred=TRUE)) %>%
collect_predictions()
#> ! Fold01: preprocessor 1/1, model 1/1: 5 columns were requested but there were 4 predictors in the data. 4 will...
#> ! Fold02: preprocessor 1/1, model 1/1: 5 columns were requested but there were 4 predictors in the data. 4 will...
#> ! Fold03: preprocessor 1/1, model 1/1: 5 columns were requested but there were 4 predictors in the data. 4 will...
#> ! Fold04: preprocessor 1/1, model 1/1: 5 columns were requested but there were 4 predictors in the data. 4 will...
#> ! Fold05: preprocessor 1/1, model 1/1: 5 columns were requested but there were 4 predictors in the data. 4 will...
#> ! Fold06: preprocessor 1/1, model 1/1: 5 columns were requested but there were 4 predictors in the data. 4 will...
#> ! Fold07: preprocessor 1/1, model 1/1: 5 columns were requested but there were 4 predictors in the data. 4 will...
#> ! Fold08: preprocessor 1/1, model 1/1: 5 columns were requested but there were 4 predictors in the data. 4 will...
#> ! Fold09: preprocessor 1/1, model 1/1: 5 columns were requested but there were 4 predictors in the data. 4 will...
#> ! Fold10: preprocessor 1/1, model 1/1: 5 columns were requested but there were 4 predictors in the data. 4 will...
# incorrect roc
rf_training_pred %>%
roc_curve(truth=Species,.pred_setosa,.pred_virginica,.pred_versicolor) %>%
autoplot(rf_training_roc)
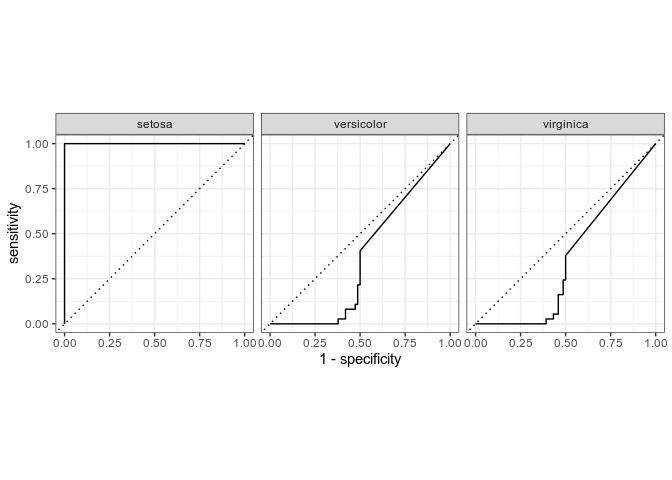
# correct roc
rf_training_pred %>%
roc_curve(truth=Species,.pred_setosa,.pred_versicolor,.pred_virginica) %>%
autoplot(rf_training_roc)
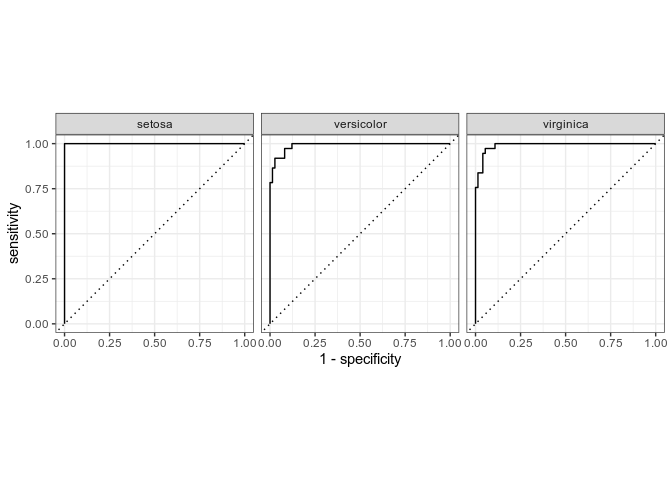
Created on 2022-09-22 by the reprex package (v2.0.1)
Session info
sessioninfo::session_info()
#> ─ Session info ───────────────────────────────────────────────────────────────
#> setting value
#> version R version 4.1.0 (2021-05-18)
#> os Ubuntu 20.04.5 LTS
#> system x86_64, linux-gnu
#> ui X11
#> language en_GB:en
#> collate en_GB.UTF-8
#> ctype en_GB.UTF-8
#> tz Europe/Stockholm
#> date 2022-09-22
#> pandoc 2.18 @ /usr/lib/rstudio/bin/quarto/bin/tools/ (via rmarkdown)
#>
#> ─ Packages ───────────────────────────────────────────────────────────────────
#> package * version date (UTC) lib source
#> assertthat 0.2.1 2019-03-21 [1] CRAN (R 4.1.0)
#> backports 1.4.1 2021-12-13 [1] CRAN (R 4.1.0)
#> broom * 1.0.0 2022-07-01 [1] CRAN (R 4.1.0)
#> class 7.3-20 2022-01-13 [1] CRAN (R 4.1.0)
#> cli 3.3.0 2022-04-25 [1] CRAN (R 4.1.0)
#> codetools 0.2-18 2020-11-04 [1] CRAN (R 4.1.0)
#> colorspace 2.0-3 2022-02-21 [1] CRAN (R 4.1.0)
#> crayon 1.5.1 2022-03-26 [1] CRAN (R 4.1.0)
#> curl 4.3.2 2021-06-23 [1] CRAN (R 4.1.0)
#> DBI 1.1.3 2022-06-18 [1] CRAN (R 4.1.0)
#> dials * 1.0.0 2022-06-14 [1] CRAN (R 4.1.0)
#> DiceDesign 1.9 2021-02-13 [1] CRAN (R 4.1.0)
#> digest 0.6.29 2021-12-01 [1] CRAN (R 4.1.0)
#> dplyr * 1.0.9 2022-04-28 [1] CRAN (R 4.1.0)
#> ellipsis 0.3.2 2021-04-29 [1] CRAN (R 4.1.0)
#> evaluate 0.16 2022-08-09 [1] CRAN (R 4.1.0)
#> fansi 1.0.3 2022-03-24 [1] CRAN (R 4.1.0)
#> farver 2.1.1 2022-07-06 [1] CRAN (R 4.1.0)
#> fastmap 1.1.0 2021-01-25 [1] CRAN (R 4.1.0)
#> foreach 1.5.2 2022-02-02 [1] CRAN (R 4.1.0)
#> fs 1.5.2 2021-12-08 [1] CRAN (R 4.1.0)
#> furrr 0.3.1 2022-08-15 [1] CRAN (R 4.1.0)
#> future 1.27.0 2022-07-22 [1] CRAN (R 4.1.0)
#> future.apply 1.9.0 2022-04-25 [1] CRAN (R 4.1.0)
#> generics 0.1.3 2022-07-05 [1] CRAN (R 4.1.0)
#> ggplot2 * 3.3.6 2022-05-03 [1] CRAN (R 4.1.0)
#> globals 0.16.0 2022-08-05 [1] CRAN (R 4.1.0)
#> glue 1.6.2 2022-02-24 [1] CRAN (R 4.1.0)
#> gower 1.0.0 2022-02-03 [1] CRAN (R 4.1.0)
#> GPfit 1.0-8 2019-02-08 [1] CRAN (R 4.1.0)
#> gtable 0.3.0 2019-03-25 [1] CRAN (R 4.1.0)
#> hardhat 1.2.0 2022-06-30 [1] CRAN (R 4.1.0)
#> highr 0.9 2021-04-16 [1] CRAN (R 4.1.0)
#> htmltools 0.5.3 2022-07-18 [1] CRAN (R 4.1.0)
#> httr 1.4.3 2022-05-04 [1] CRAN (R 4.1.0)
#> infer * 1.0.3 2022-08-22 [1] CRAN (R 4.1.0)
#> ipred 0.9-13 2022-06-02 [1] CRAN (R 4.1.0)
#> iterators 1.0.14 2022-02-05 [1] CRAN (R 4.1.0)
#> knitr 1.39 2022-04-26 [1] CRAN (R 4.1.0)
#> labeling 0.4.2 2020-10-20 [1] CRAN (R 4.1.0)
#> lattice 0.20-45 2021-09-22 [1] CRAN (R 4.1.0)
#> lava 1.6.10 2021-09-02 [1] CRAN (R 4.1.0)
#> lhs 1.1.5 2022-03-22 [1] CRAN (R 4.1.0)
#> lifecycle 1.0.1 2021-09-24 [1] CRAN (R 4.1.0)
#> listenv 0.8.0 2019-12-05 [1] CRAN (R 4.1.0)
#> lubridate 1.8.0 2021-10-07 [1] CRAN (R 4.1.0)
#> magrittr 2.0.3 2022-03-30 [1] CRAN (R 4.1.0)
#> MASS 7.3-58.1 2022-08-03 [1] CRAN (R 4.1.0)
#> Matrix 1.4-1 2022-03-23 [1] CRAN (R 4.1.0)
#> mime 0.12 2021-09-28 [1] CRAN (R 4.1.0)
#> modeldata * 1.0.1 2022-09-06 [1] CRAN (R 4.1.0)
#> munsell 0.5.0 2018-06-12 [1] CRAN (R 4.1.0)
#> nnet 7.3-17 2022-01-13 [1] CRAN (R 4.1.0)
#> parallelly 1.32.1 2022-07-21 [1] CRAN (R 4.1.0)
#> parsnip * 1.0.1 2022-08-18 [1] CRAN (R 4.1.0)
#> pillar 1.8.0 2022-07-18 [1] CRAN (R 4.1.0)
#> pkgconfig 2.0.3 2019-09-22 [1] CRAN (R 4.1.0)
#> prodlim 2019.11.13 2019-11-17 [1] CRAN (R 4.1.0)
#> purrr * 0.3.4 2020-04-17 [1] CRAN (R 4.1.0)
#> R.cache 0.16.0 2022-07-21 [1] CRAN (R 4.1.0)
#> R.methodsS3 1.8.2 2022-06-13 [1] CRAN (R 4.1.0)
#> R.oo 1.25.0 2022-06-12 [1] CRAN (R 4.1.0)
#> R.utils 2.12.0 2022-06-28 [1] CRAN (R 4.1.0)
#> R6 2.5.1 2021-08-19 [1] CRAN (R 4.1.0)
#> ranger * 0.14.1 2022-06-18 [1] CRAN (R 4.1.0)
#> Rcpp 1.0.9 2022-07-08 [1] CRAN (R 4.1.0)
#> recipes * 1.0.1 2022-07-07 [1] CRAN (R 4.1.0)
#> reprex 2.0.1 2021-08-05 [1] CRAN (R 4.1.0)
#> rlang 1.0.4 2022-07-12 [1] CRAN (R 4.1.0)
#> rmarkdown 2.14 2022-04-25 [1] CRAN (R 4.1.0)
#> rpart 4.1.16 2022-01-24 [1] CRAN (R 4.1.0)
#> rsample * 1.1.0 2022-08-08 [1] CRAN (R 4.1.0)
#> rstudioapi 0.13 2020-11-12 [1] CRAN (R 4.1.0)
#> scales * 1.2.0 2022-04-13 [1] CRAN (R 4.1.0)
#> sessioninfo 1.2.2 2021-12-06 [1] CRAN (R 4.1.0)
#> stringi 1.7.8 2022-07-11 [1] CRAN (R 4.1.0)
#> stringr 1.4.0 2019-02-10 [1] CRAN (R 4.1.0)
#> styler 1.7.0 2022-03-13 [1] CRAN (R 4.1.0)
#> survival 3.4-0 2022-08-09 [1] CRAN (R 4.1.0)
#> tibble * 3.1.8 2022-07-22 [1] CRAN (R 4.1.0)
#> tidymodels * 1.0.0 2022-07-13 [1] CRAN (R 4.1.0)
#> tidyr * 1.2.0 2022-02-01 [1] CRAN (R 4.1.0)
#> tidyselect 1.1.2 2022-02-21 [1] CRAN (R 4.1.0)
#> timeDate 4021.104 2022-07-19 [1] CRAN (R 4.1.0)
#> tune * 1.0.0 2022-07-07 [1] CRAN (R 4.1.0)
#> utf8 1.2.2 2021-07-24 [1] CRAN (R 4.1.0)
#> vctrs 0.4.1 2022-04-13 [1] CRAN (R 4.1.0)
#> withr 2.5.0 2022-03-03 [1] CRAN (R 4.1.0)
#> workflows * 1.0.0 2022-07-05 [1] CRAN (R 4.1.0)
#> workflowsets * 1.0.0 2022-07-12 [1] CRAN (R 4.1.0)
#> xfun 0.32 2022-08-10 [1] CRAN (R 4.1.0)
#> xml2 1.3.3 2021-11-30 [1] CRAN (R 4.1.0)
#> yaml 2.3.5 2022-02-21 [1] CRAN (R 4.1.0)
#> yardstick * 1.1.0 2022-09-07 [1] CRAN (R 4.1.0)
#>
#>
#> ──────────────────────────────────────────────────────────────────────────────