This is a good discussion to continue @startz (my fellow Richard) because I've revisited the linear probability model (PLM). I first saw it a few years ago in an econometric's paper on gender discrimination in the financial industry and was not convinced by the authors' defense of the methodology.
The first example given in the cited text is one in which I had a lot of domain knowledge before 2009, so it serves as a good motivating example. The data to be analyzed concerns mortgage loan application approvals.
---------------------------------------------------------
Describe HMDA (data.frame):
data frame: 2380 obs. of 14 variables
2380 complete cases (100.0%)
Nr ColName Class NAs Levels
1 deny factor . (2): 1-no, 2-yes
2 pirat numeric .
3 hirat numeric .
4 lvrat numeric .
5 chist factor . (6): 1-1, 2-2, 3-3,
4-4, 5-5, ...
6 mhist factor . (4): 1-1, 2-2, 3-3, 4-4
7 phist factor . (2): 1-no, 2-yes
8 unemp numeric .
9 selfemp factor . (2): 1-no, 2-yes
10 insurance factor . (2): 1-no, 2-yes
11 condomin factor . (2): 1-no, 2-yes
12 afam factor . (2): 1-no, 2-yes
13 single factor . (2): 1-no, 2-yes
14 hschool factor . (2): 1-no, 2-yes
The response (dependent) variable (or regressand) Y is deny
, so a value of 0 indicates loan approval (deny
is FALSE, 0) while a value of 1
is disapproval.
The treatment (independent) variable (or regressor) X is pirat
called P/I ratio
, the ratio of scheduled payments of principal and interest on the mortgage loan to income.
The PLM model claims that Y = \beta_0 + \beta_1 * X - u where
\beta_0 is the y-intercept of the regression line
\beta_1 is the slope of the regression line
u is an error term
Earlier in the text, u is explained
the error term u\dots captures additional random effects \dots all the differences between the regression line and the actual observed data. Beside pure randomness, these deviations could also arise from measurement errors or, as will be discussed later, could be the consequence of leaving out other factors that are relevant in explaining the dependent variable.
u, accordingly, may as well stand for Universal Fudge Factor
u is not a term considered necessary in ordinary least square regression, and for good reason. If the question that a linear regression is supposed to answer is what is the probability of observing Y in the presence of X and the answer is 2.54 + 1.39x, plus or minus some other stuff, that is not a very satisfactory state of affairs.
Let's start by ticking off one other consideration. The formula is linear in its parameters, not Y = \beta_0 + \beta_1x^2 + u.
So, here's the example adapted from the text:
library(ggplot2)
data("HMDA",package = "AER")
# inspect the data
DescTools::Desc(HMDA, plotit = TRUE)
#> ------------------------------------------------------------------------------
#> Describe HMDA (data.frame):
#>
#> data frame: 2380 obs. of 14 variables
#> 2380 complete cases (100.0%)
#>
#> Nr ColName Class NAs Levels
#> 1 deny factor . (2): 1-no, 2-yes
#> 2 pirat numeric .
#> 3 hirat numeric .
#> 4 lvrat numeric .
#> 5 chist factor . (6): 1-1, 2-2, 3-3, 4-4, 5-5, ...
#> 6 mhist factor . (4): 1-1, 2-2, 3-3, 4-4
#> 7 phist factor . (2): 1-no, 2-yes
#> 8 unemp numeric .
#> 9 selfemp factor . (2): 1-no, 2-yes
#> 10 insurance factor . (2): 1-no, 2-yes
#> 11 condomin factor . (2): 1-no, 2-yes
#> 12 afam factor . (2): 1-no, 2-yes
#> 13 single factor . (2): 1-no, 2-yes
#> 14 hschool factor . (2): 1-no, 2-yes
#>
#>
#> ------------------------------------------------------------------------------
#> 1 - deny (factor - dichotomous)
#>
#> length n NAs unique
#> 2'380 2'380 0 2
#> 100.0% 0.0%
#>
#> freq perc lci.95 uci.95'
#> no 2'095 88.0% 86.7% 89.3%
#> yes 285 12.0% 10.7% 13.3%
#>
#> ' 95%-CI (Wilson)
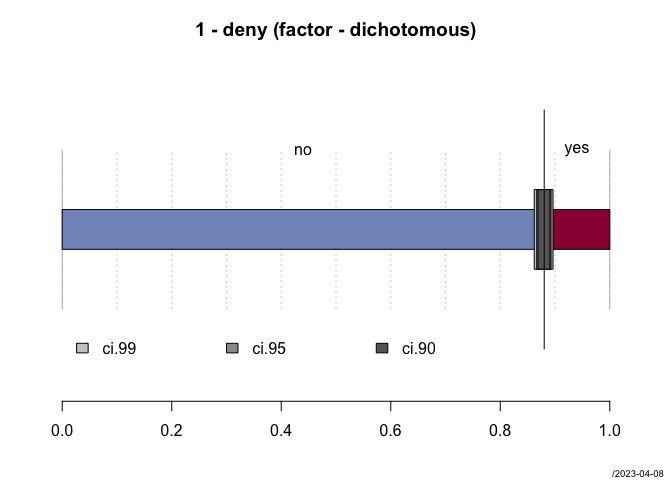
#> ------------------------------------------------------------------------------
#> 2 - pirat (numeric)
#>
#> length n NAs unique 0s mean meanCI'
#> 2'380 2'380 0 519 1 0.3308 0.3265
#> 100.0% 0.0% 0.0% 0.3351
#>
#> .05 .10 .25 median .75 .90 .95
#> 0.1900 0.2300 0.2800 0.3300 0.3700 0.4100 0.4401
#>
#> range sd vcoef mad IQR skew kurt
#> 3.0000 0.1073 0.3242 0.0608 0.0900 8.0816 174.0668
#>
#> lowest : 0.0, 0.04 (2), 0.056, 0.0699, 0.07 (3)
#> highest: 0.95, 1.16, 1.28, 1.42 (2), 3.0
#>
#> ' 95%-CI (classic)
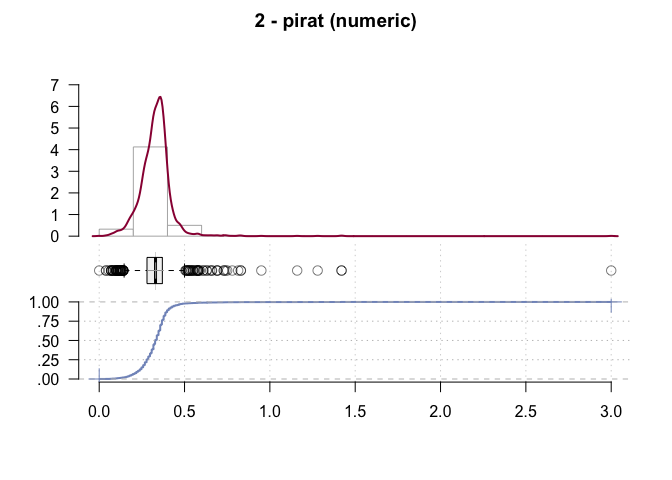
#> ------------------------------------------------------------------------------
#> 3 - hirat (numeric)
#>
#> length n NAs unique 0s mean meanCI'
#> 2'380 2'380 0 500 3 0.255346 0.251461
#> 100.0% 0.0% 0.1% 0.259231
#>
#> .05 .10 .25 median .75 .90 .95
#> 0.120000 0.160000 0.214000 0.260000 0.298825 0.330000 0.350000
#>
#> range sd vcoef mad IQR skew kurt
#> 3.000000 0.096656 0.378528 0.059304 0.084825 10.306709 277.842289
#>
#> lowest : 0.0 (3), 0.00085, 0.01 (2), 0.02 (3), 0.028
#> highest: 0.72 (2), 0.73, 0.74, 1.1 (2), 3.0
#>
#> heap(?): remarkable frequency (6.0%) for the mode(s) (= 0.28)
#>
#> ' 95%-CI (classic)
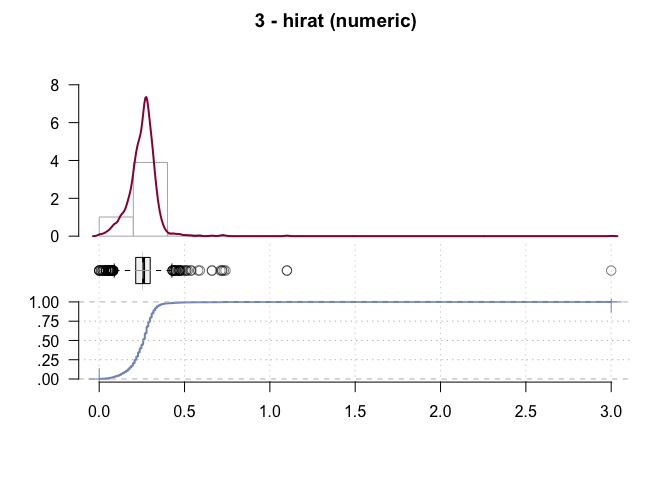
#> ------------------------------------------------------------------------------
#> 4 - lvrat (numeric)
#>
#> length n NAs unique 0s mean meanCI'
#> 2'380 2'380 0 1'537 0 0.7377759 0.7305909
#> 100.0% 0.0% 0.0% 0.7449609
#>
#> .05 .10 .25 median .75 .90 .95
#> 0.3797828 0.4895612 0.6526808 0.7795364 0.8684586 0.9040000 0.9412107
#>
#> range sd vcoef mad IQR skew kurt
#> 1.9300000 0.1787510 0.2422836 0.1524358 0.2157779 -0.6013342 2.9580578
#>
#> lowest : 0.02, 0.0315107, 0.0869565, 0.1025641, 0.1096491
#> highest: 1.4651163, 1.4782609, 1.6, 1.9083333, 1.95
#>
#> heap(?): remarkable frequency (6.1%) for the mode(s) (= 0.8)
#>
#> ' 95%-CI (classic)
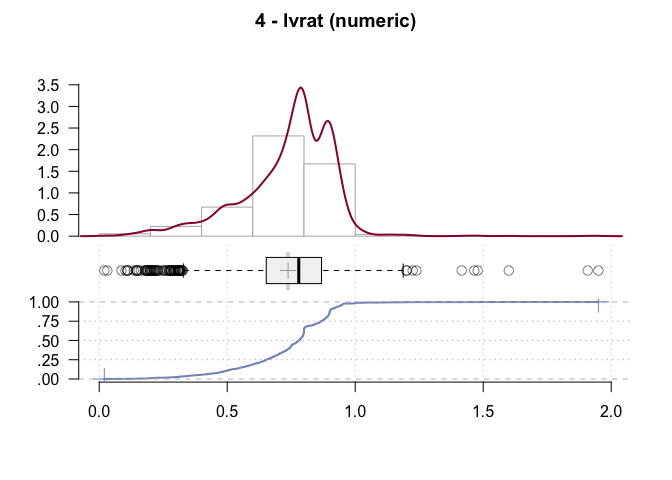
#> ------------------------------------------------------------------------------
#> 5 - chist (factor)
#>
#> length n NAs unique levels dupes
#> 2'380 2'380 0 6 6 y
#> 100.0% 0.0%
#>
#> level freq perc cumfreq cumperc
#> 1 1 1'353 56.8% 1'353 56.8%
#> 2 2 441 18.5% 1'794 75.4%
#> 3 6 201 8.4% 1'995 83.8%
#> 4 5 182 7.6% 2'177 91.5%
#> 5 3 126 5.3% 2'303 96.8%
#> 6 4 77 3.2% 2'380 100.0%
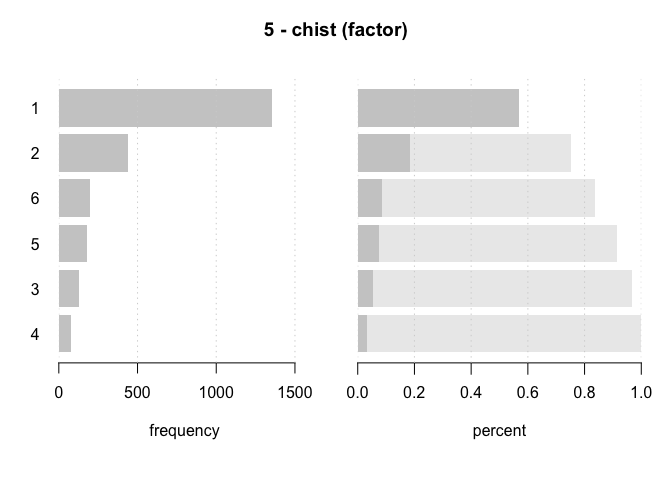
#> ------------------------------------------------------------------------------
#> 6 - mhist (factor)
#>
#> length n NAs unique levels dupes
#> 2'380 2'380 0 4 4 y
#> 100.0% 0.0%
#>
#> level freq perc cumfreq cumperc
#> 1 2 1'571 66.0% 1'571 66.0%
#> 2 1 747 31.4% 2'318 97.4%
#> 3 3 41 1.7% 2'359 99.1%
#> 4 4 21 0.9% 2'380 100.0%
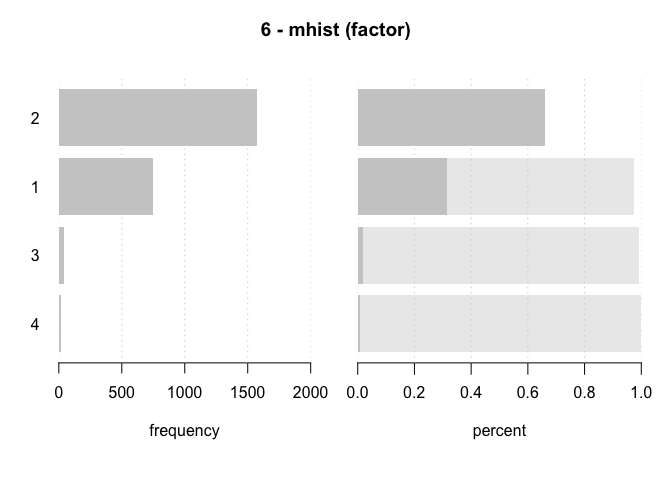
#> ------------------------------------------------------------------------------
#> 7 - phist (factor - dichotomous)
#>
#> length n NAs unique
#> 2'380 2'380 0 2
#> 100.0% 0.0%
#>
#> freq perc lci.95 uci.95'
#> no 2'205 92.6% 91.5% 93.6%
#> yes 175 7.4% 6.4% 8.5%
#>
#> ' 95%-CI (Wilson)
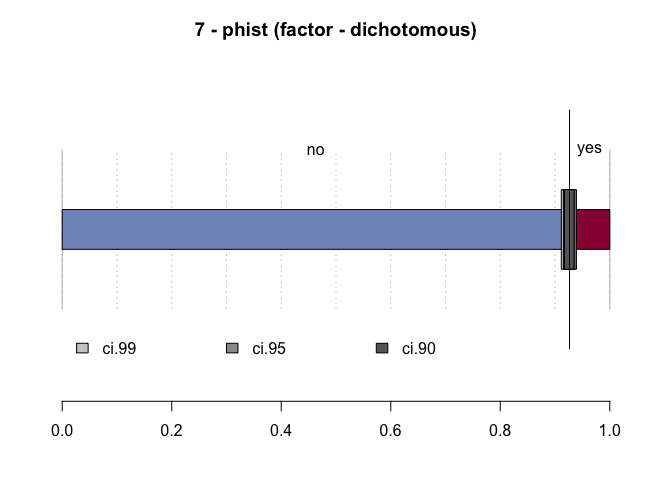
#> ------------------------------------------------------------------------------
#> 8 - unemp (numeric)
#>
#> length n NAs unique 0s mean meanCI'
#> 2'380 2'380 0 10 0 3.77 3.69
#> 100.0% 0.0% 0.0% 3.86
#>
#> .05 .10 .25 median .75 .90 .95
#> 1.80 2.00 3.10 3.20 3.90 5.30 10.60
#>
#> range sd vcoef mad IQR skew kurt
#> 8.80 2.03 0.54 0.59 0.80 2.53 5.99
#>
#>
#> value freq perc cumfreq cumperc
#> 1 1.79999995231628 217 9.1% 217 9.1%
#> 2 2 148 6.2% 365 15.3%
#> 3 3.09999990463257 275 11.6% 640 26.9%
#> 4 3.20000004768372 877 36.8% 1'517 63.7%
#> 5 3.59999990463257 174 7.3% 1'691 71.1%
#> 6 3.90000009536743 229 9.6% 1'920 80.7%
#> 7 4.30000019073486 220 9.2% 2'140 89.9%
#> 8 5.30000019073486 65 2.7% 2'205 92.6%
#> 9 8.89999961853027 16 0.7% 2'221 93.3%
#> 10 10.6000003814697 159 6.7% 2'380 100.0%
#>
#> ' 95%-CI (classic)
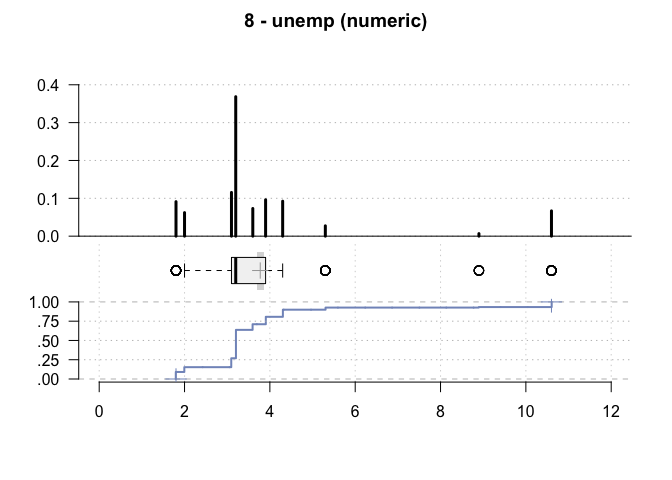
#> ------------------------------------------------------------------------------
#> 9 - selfemp (factor - dichotomous)
#>
#> length n NAs unique
#> 2'380 2'380 0 2
#> 100.0% 0.0%
#>
#> freq perc lci.95 uci.95'
#> no 2'103 88.4% 87.0% 89.6%
#> yes 277 11.6% 10.4% 13.0%
#>
#> ' 95%-CI (Wilson)
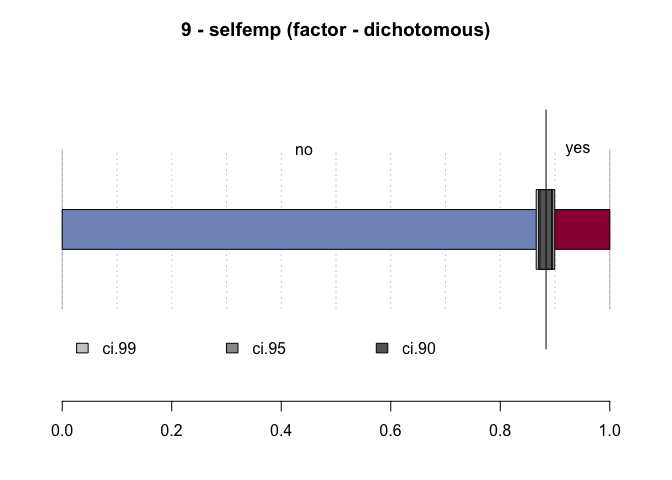
#> ------------------------------------------------------------------------------
#> 10 - insurance (factor - dichotomous)
#>
#> length n NAs unique
#> 2'380 2'380 0 2
#> 100.0% 0.0%
#>
#> freq perc lci.95 uci.95'
#> no 2'332 98.0% 97.3% 98.5%
#> yes 48 2.0% 1.5% 2.7%
#>
#> ' 95%-CI (Wilson)
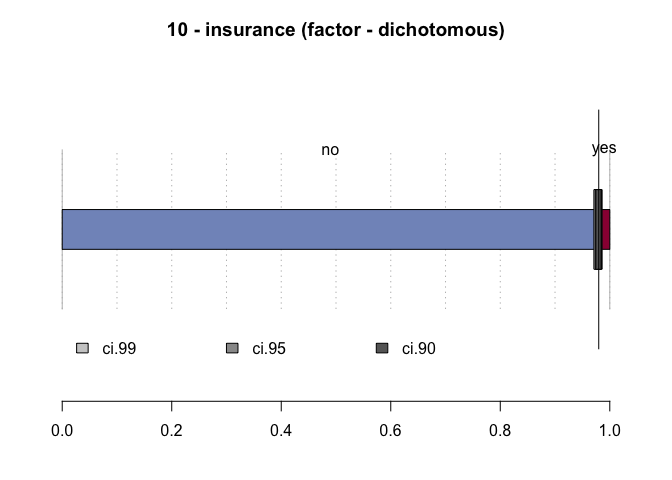
#> ------------------------------------------------------------------------------
#> 11 - condomin (factor - dichotomous)
#>
#> length n NAs unique
#> 2'380 2'380 0 2
#> 100.0% 0.0%
#>
#> freq perc lci.95 uci.95'
#> no 1'694 71.2% 69.3% 73.0%
#> yes 686 28.8% 27.0% 30.7%
#>
#> ' 95%-CI (Wilson)
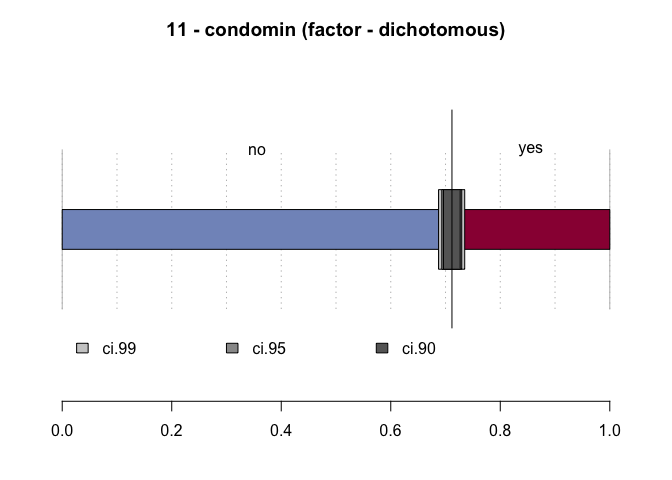
#> ------------------------------------------------------------------------------
#> 12 - afam (factor - dichotomous)
#>
#> length n NAs unique
#> 2'380 2'380 0 2
#> 100.0% 0.0%
#>
#> freq perc lci.95 uci.95'
#> no 2'041 85.8% 84.3% 87.1%
#> yes 339 14.2% 12.9% 15.7%
#>
#> ' 95%-CI (Wilson)
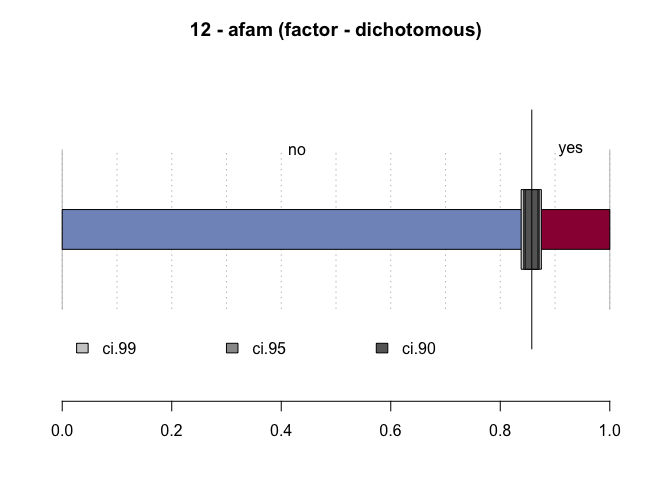
#> ------------------------------------------------------------------------------
#> 13 - single (factor - dichotomous)
#>
#> length n NAs unique
#> 2'380 2'380 0 2
#> 100.0% 0.0%
#>
#> freq perc lci.95 uci.95'
#> no 1'444 60.7% 58.7% 62.6%
#> yes 936 39.3% 37.4% 41.3%
#>
#> ' 95%-CI (Wilson)
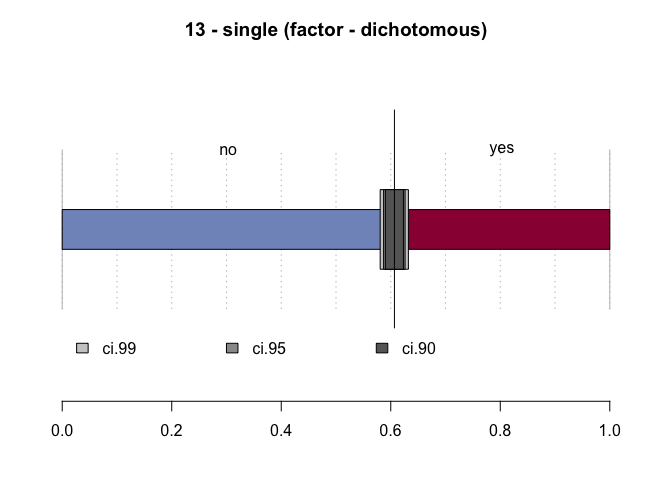
#> ------------------------------------------------------------------------------
#> 14 - hschool (factor - dichotomous)
#>
#> length n NAs unique
#> 2'380 2'380 0 2
#> 100.0% 0.0%
#>
#> freq perc lci.95 uci.95'
#> no 39 1.6% 1.2% 2.2%
#> yes 2'341 98.4% 97.8% 98.8%
#>
#> ' 95%-CI (Wilson)
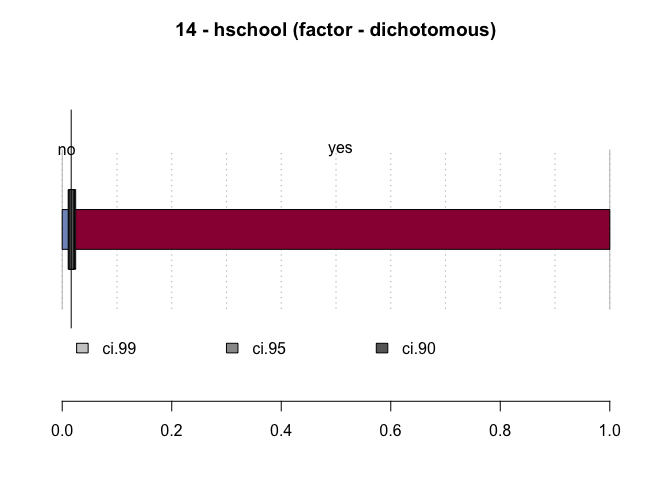
# subset the variables to be used in the example
d <- HMDA[1:2]
# convert factor to numeric 1/0
d[,1] <- as.numeric(d[,1]) - 1
# show them
d |> ggplot(aes(pirat,deny)) +
geom_point() +
geom_smooth(method = "lm") +
theme_minimal()
#> `geom_smooth()` using formula = 'y ~ x'
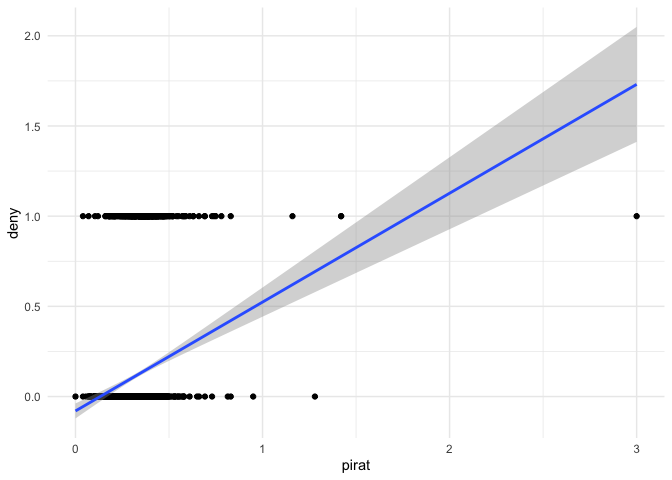
# model
fit <- lm(deny ~ pirat,d)
summary(fit)
#>
#> Call:
#> lm(formula = deny ~ pirat, data = d)
#>
#> Residuals:
#> Min 1Q Median 3Q Max
#> -0.73070 -0.13736 -0.11322 -0.07097 1.05577
#>
#> Coefficients:
#> Estimate Std. Error t value Pr(>|t|)
#> (Intercept) -0.07991 0.02116 -3.777 0.000163 ***
#> pirat 0.60353 0.06084 9.920 < 2e-16 ***
#> ---
#> Signif. codes: 0 '***' 0.001 '**' 0.01 '*' 0.05 '.' 0.1 ' ' 1
#>
#> Residual standard error: 0.3183 on 2378 degrees of freedom
#> Multiple R-squared: 0.03974, Adjusted R-squared: 0.03933
#> F-statistic: 98.41 on 1 and 2378 DF, p-value: < 2.2e-16
# plot from text
plot(x = d$pirat,
y = d$deny,
main = "Scatterplot Mortgage Application Denial and the Payment-to-Income Ratio",
xlab = "P/I ratio",
ylab = "Deny",
pch = 20,
ylim = c(-0.4, 1.4),
cex.main = 0.8)
# add horizontal dashed lines and text
abline(h = 1, lty = 2, col = "darkred")
abline(h = 0, lty = 2, col = "darkred")
text(2.5, 0.9, cex = 0.8, "Mortgage denied")
text(2.5, -0.1, cex= 0.8, "Mortgage approved")
# add the estimated regression line
abline(fit,
lwd = 1.8,
col = "steelblue")
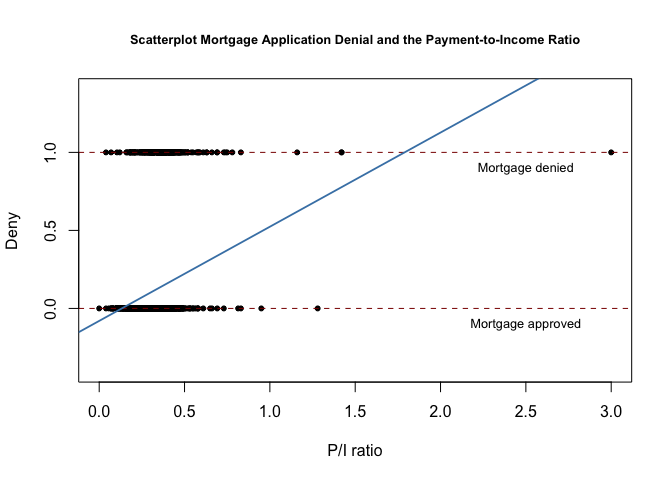
# print robust coefficient summary
coeftest(fit, vcov. = vcovHC, type = "HC1")
#> Error in coeftest(fit, vcov. = vcovHC, type = "HC1"): could not find function "coeftest"
# standard plots (not in text)
par(mfrow = c(2,2))
plot(fit)
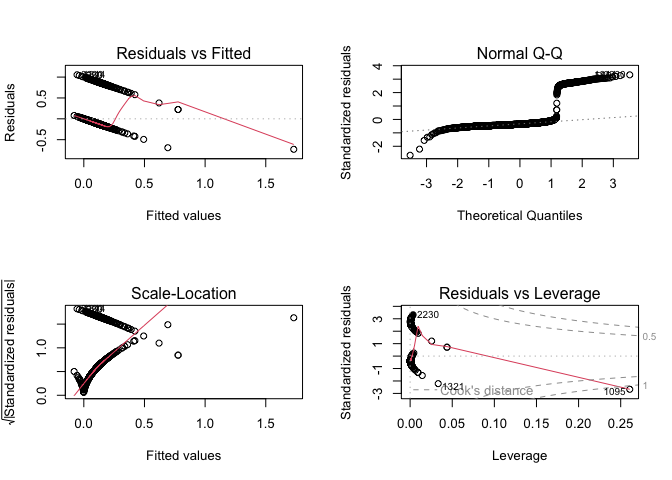
The claim is that the model be interpreted as evidence that each 1% (0.01) increase in the variable pirat
increases the probability of denial of a loan application by 0.00604, or 0.6%.
Is that useful information in light of the data? Time for a reality check. There's some bad data
> d[d[2] > 1,]
deny pirat
621 1 1.16
1095 1 3.00
1321 0 1.28
1928 1 1.42
1929 1 1.42
Even during the height of the mortgage lending craze loans with payment terms amounting to 128% of income were not extended. And, of course the other applications above 100% were rejected. In fact, the "back-end debt-to-income ratio was used and it added to payments of principal and interest credit card, auto loan, student loan and all other disclosed debt. The soft cut-off for that ratio was 38% and of the last 125,000 loan summaries I looked at 55% was the highest I ever saw.
This matters because of the reality distortion field that may be generated. To test, I subset to exclude values greater than 50%. I found that doing so approximately doubled the probability of denial of a loan application for each 1% increase in the P/I ratio. A cautionary tale the moral of which is that while the data may not lie, they are entirely capable of deceit.
Several points to note on the standard diagnostic plot
- As noted in the text R^2 has no interpretation
- Although linear in the parameters, the residuals vs. fitted plot doesn't cluster around zero
- QQ plot shows residuals are not normally distributed
- Scale-location shows heteroskedascity to the max
- The outliers in residuals vs. leverage probably indicate the influence of the anomolously large values of the variable
pirat
The model also predicts probabilities of denial in excess of 1.
(Left as an exercise—divide the data into training and test sets and compare predictions of the test data to the observed results and compare RMSE to just the proportions in a two-way contingency table.)
The two principal arguments advanced in the case of the linear probability model are simplicity and robustness. We no longer use desk calculators, so the first argument can be dismissed out of hand. Robustness cannot compensate for a non-normal distribution of residuals. Brushed aside is the usually untenable assumption that the u term can be ignored in the calculations and usable results can be obtained by assuming away not only any influence of other variables but their existence also and treating the model as entirely endogenous. As my country cousins say that dog won't hunt.
And that's even before confronting the principal difficulty in the case presented by the OP, which is that not only is the response variable binary, so is the variable being regressed against.
Just. Don't. Go. There.