Representative is a judgment call. The tools to aid in forming the judgment vary by domain and the nature of the data. I'd suggest approaching it from a functional analytic framework. f(x) = y. where x is the object to hand, y is the object desired and f is the function to apply to x to return y.
In this case you have two tables, one with n = 400, representing the demographic variables collected on the responding subjects and another with n = 17000 with the same variables for the population of interest. By design, the first is a subset of the second. By what measure(s) do the two tables differ apart from n?
If you have the 17,000 records, and not just their summary statistics, measures of central tendency can be compared and an assessment as to whether the population and subset have means that differ to a greater tolerance than would be expected solely as a result of random variation. A similar estimate can be made for differences in the median. Distribution differences can be considered.
One way to do those three assessments for a variable age
, for example would a notched boxplot to provide a visual comparison of mean, median and the prevalence of outliers. That may be sufficient clearly to show that while the population has a mean age of 50, a median age of 37, and few instances of representatives above and below 1.5 times the difference between the 25th and 75th percentile (the interquartile distance), the surveyed population has a mean of 30, a median of 27 and noticeable presence of outliers in the upper range. It would be possible to put a finer gloss on that, but its a clear difference that stands out clearly. On the other hand, if they look closer, there are tests to put a number on it.
Beginning with the variables that, in your assessment, are most relevant to the survey responses, an exploratory data analysis with boxplots for the continuous variables would be a good place to start.
On the other hand, for categorical and ordinal variables, different tools are needed. Contingency tables are helpful. For example,
library(vcd)
#> Loading required package: grid
HairEyeColor
#> , , Sex = Male
#>
#> Eye
#> Hair Brown Blue Hazel Green
#> Black 32 11 10 3
#> Brown 53 50 25 15
#> Red 10 10 7 7
#> Blond 3 30 5 8
#>
#> , , Sex = Female
#>
#> Eye
#> Hair Brown Blue Hazel Green
#> Black 36 9 5 2
#> Brown 66 34 29 14
#> Red 16 7 7 7
#> Blond 4 64 5 8
(hec <- margin.table(HairEyeColor, 2:1))
#> Hair
#> Eye Black Brown Red Blond
#> Brown 68 119 26 7
#> Blue 20 84 17 94
#> Hazel 15 54 14 10
#> Green 5 29 14 16
tile(hec)
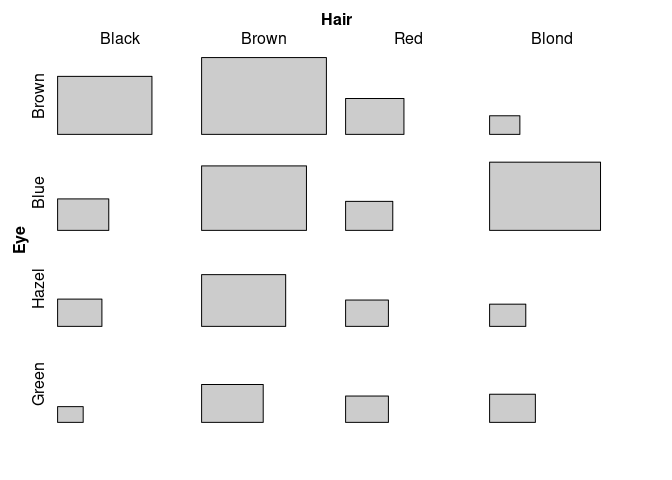
Created on 2022-11-11 by the reprex package (v2.0.1)
Finally, you could compare the n = 400 with repeated random samples of the same size from the n = 17000 in terms of how different or not the observed demographics are to what would have been obtained by random sampling.